Data-Driven Policy Design: Leveraging the iSDG Model for Informed Development
- fernandoredivo
- Feb 9, 2024
- 3 min read
Updated: Jun 6, 2024
The United Nations has strongly highlighted the importance of strategically leveraging data in sustainable development. They state an average return of $32 for every $1 invested in strengthening data systems in low and middle income countries, a concept referred to as the 'data dividend'. Furthermore, the Cape Town Global Action Plan for Sustainable Development Data, adopted by the UN Statistical Commission, underscores the necessity of modernizing national statistical systems. This plan is crucial for meeting the increasing data demands of the 2030 Agenda for Sustainable Development and for improving the quality, accessibility, and reliability of data in developing countries, thereby playing a pivotal role in achieving the Sustainable Development Goals.
The key challenge, however, is the effective transformation of this data into actionable policies. The Integrated Sustainable Development Goals (iSDG) model, developed by the Millennium Institute, is engineered to address this challenge. It converts large amounts of data into insights for sustainable development, offering comprehensible information for policymakers and stakeholders. This approach underscores the importance of not only gathering data but also presenting it in a way that is understandable and actionable
The iSDG model is a comprehensive simulation tool that helps policymakers to assess the potential impact of various policies on achieving the Sustainable Development Goals (SDGs). It integrates economic, social, and environmental factors into a cohesive framework, allowing for the analysis of complex interdependencies and long-term consequences of decisions.
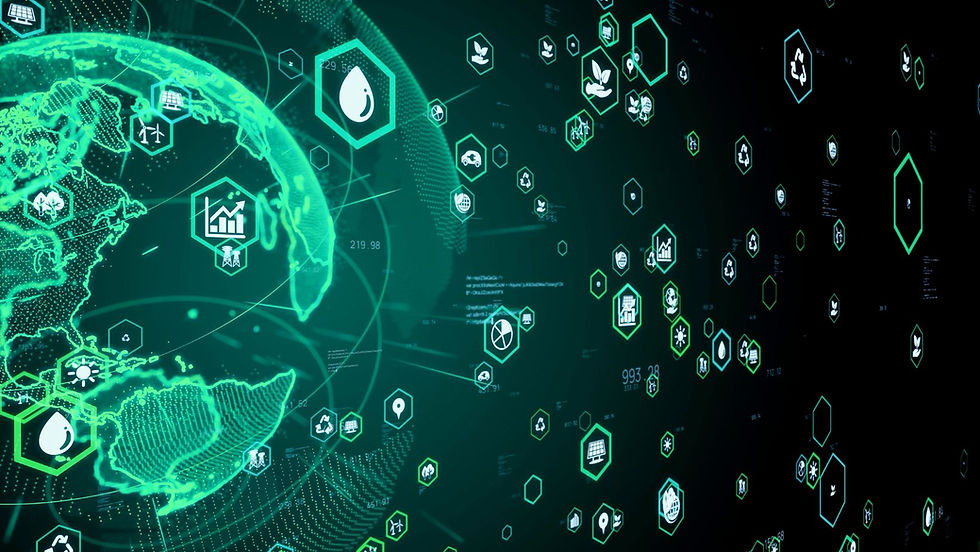
Data Integration in the iSDG Model
A critical component of the iSDG model's efficacy is its data integration process. This involves collecting data from organizations responsible for global monitoring as SDG custodian agencies, such as the World Bank, FAO, and UNSTATS. These organizations play a pivotal role in providing reliable data essential for monitoring progress towards the Sustainable Development Goals. For detailed information on the international organizations responsible for global monitoring for each SDG indicator, you can consult the SDG Indicators Metadata repository. Other international datasets, as well as national databases are also included in our data integration process.
The Millennium Institute's approach to data handling for the iSDG model involves two steps:
Evaluating Indicator Relevancy: We assess the relevance of indicators for modeling and policy analysis. This involves analyzing and cross-referencing methodological background documents, conducting literature reviews, and performing tests to ensure the indicators align with the model's objectives.
Assessing Data Quality: This step is conducted on a country-by-country basis, both independently and in collaboration with appointed government representatives. It ensures that the data accurately reflects the dynamics and objectives specific to each country, which is crucial for the iSDG model to simulate real-world scenarios effectively.
This structured approach, particularly our collaboration with government representatives, is a vital component of the data selection and integration process in the iSDG model.
Simulation vs. Real World Data
Data is the backbone of the iSDG model, essential for aligning simulations with historical development trends and enhancing accuracy. Calibrating the model against historical data is an important step, reinforcing the model's validity and fine-tuning it for policy assessment.
The graph from our Angola project exemplifies the iSDG model's application. It presents historical data alongside simulations of a 'business as usual' scenario and a policy-intervention scenario, offering a comparison of different developmental pathways.
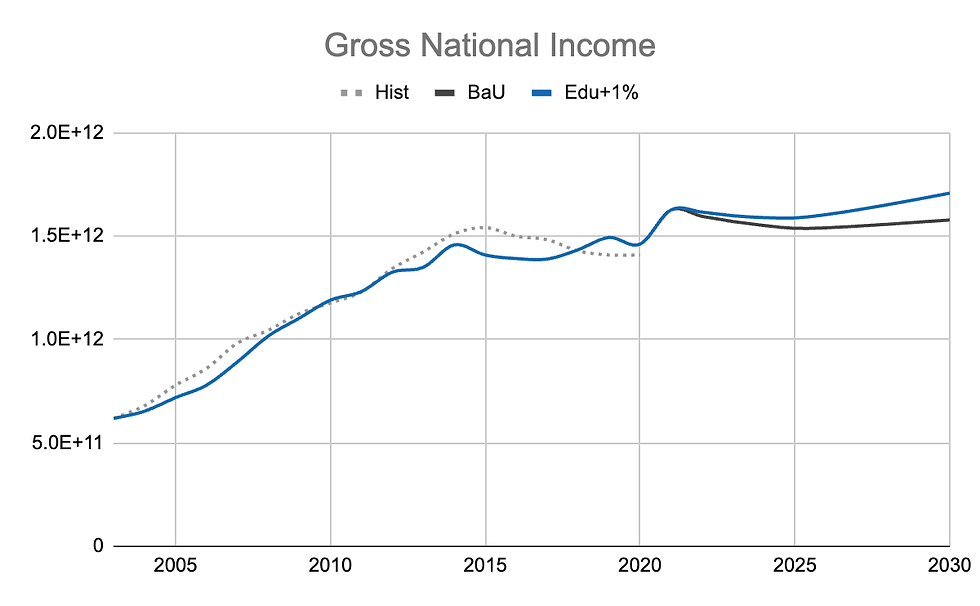
The above graph illustrates the close alignment between the simulated outputs and real-world historical data. The 'Business-as-Usual' scenario projects the trajectory of Angola's Gross National Income (GNI) absent any new policy interventions. Conversely, the 'Edu+1%' scenario simulates the effects of increasing general education funding by an additional 1% of the nation's GDP annually — a supplementary allocation beyond the current government expenditure.
The data indicates a notable return on educational investments. Specifically, the projection suggests that with a 1% annual increase in education investment, Angola's GNI could rise by an estimated 130 Billion Kwanzas (equivalent to approximately 260 million USD) by the year 2030. While the broader implications of this educational policy extend across various sectors, for the purposes of this analysis, the focus is confined to the GNI to maintain the article's clarity.
In summary, the iSDG model exemplifies the utility of data-driven methodologies for policy formulation. It translates complex data into clear visual insights, thereby providing policymakers with a representation of both the present conditions and prospective developmental outcomes. This methodology is instrumental in furthering the Sustainable Development Goals, providing a data-rich foundation for national development strategies.
Comments